Deep Textures
Using Machine Learning to create unexpected materials with textures and normal data.
Results:
Browse / Download 150 textures [google drive]
Here a curated selection of specially beautiful textures and normal maps
Play / Models released at Runway
Motivation:
Having extensively used CAD & 3D software packages as part of my product designer practice, I personally find the task of creating new materials tedious and expectable, without a surprise factor. On the other and, with my playing with GANs and image-based ML tools has a discovery factor that keeps me engaged with rewards of nice findings while exploring the unknown.
So, how could we create new 3D materials using GANs?
Proof of concept:
In order to test the look & feel of ML generated 3D materials, I ran a collection of 1000 Portuguese Azulejo Tiles images through StyleGAN to generate visually similar tiles. The results are surprisingly nice, so this seems to be a good direction.
Next step was to add depth to those generated images.
3D packages can read BW data and render it as height transformation (bump map), if this is done with the same image used as texture, results are ok but not accurate, as different colours with similar saturation will show with the same height :(.
So, I had to find translation tool to identify/segment which part of the tiles are embossed or extruded to add some height detail.
To add depth to those tiles, I ran some of them through DenseDepth model, and I got some interpertations of depth as grayscale images, not accurate probably because the model is trained with spatial scenes (Indoor and outdoor), but the results were beautiful and interesting.
Approach
The initial idea is to source a dataset composed of texture images (diffuse) and their corresponding normal map (a technique used for faking the lighting of bumps and dents. Normal maps are commonly stored as regular RGB images where the RGB components correspond to the X, Y, and Z coordinates, respectively, of the surface normal).
And then ran those images through StyleGAN.
It would ideal be to have two StyleGAN's to talk to each other while learning, so they'd know which normal-map relates to which texture... But doing that is beyond my current knowledge.
A workaround could be to stitch/merge both Diffuse + Normal textures into a single image, and ran it through the StyleGAN, with the hope that it would understand that there are two different and corresponding sides of each image, and thus, generate images with both sides... for later splitting them in order to get the generated diffuse and the corresponding generated normal.
But first, I need to see if the model is good at generating diffuse texture images from a highly diverse dataset containign bricks, fur, wood, foam... (probably yes, as it did with the Azulejo, but needs to be tested).
Diffuse Texture generation
The Dataset chosen is Describable Textures Dataset (DTD) a texture database, consisting of 5640 images, organized according to a list of 47 terms (categories) inspired from human perception. There are 120 images for each category.
The results are surprisingly consistent and rich, considering that some categories have confusing textures that are not “full-frame” but appear in objects or in context. i.e: “freckles”, “hair” or “foam”.
Describable Textures Dataset (DTD)
Normal Map generation
Finding a good dataset of normal-maps has been challenging, since there's an abundance of textures for sale but a scarcity of freely accessible ones. Initially I attempted to scrape some sites containing free textures, but it required too much effort to collect few hundred textures.
A first test was done with the dataset provided by the Single-Image SVBRDF Capture with a Rendering-Aware Deep Network project.
From this [85GB zipped] dataset I cropped and isolated a subset of 2700 normal map images to feed the StyleGAN training.
Dataset: Normal Maps
StyleGAN was able to understand what makes a normal map, and it generates consistent images, with the colors correctly placed.
I then ran the generated normal maps through blender to see how they drive light within a 3D environment.
Diffuse + Normal Map single image StyleGAN training.
Now I knew that StyleGAN was capable of generating nice textures and nice normal maps independently, but I needed the normal maps to correlate to the diffuse information. Since I wasn’t able to make two stylegans talk to each other while training, I opted for composing a dataset containing the diffuse and the normal map within a single image.
This time I choose cc0textures which has a good repository of PBR textures and provides a csv information that can be used to download the desired files via wget.
Dataset: 790 images
The generated images are beautiful and they work as expected.
StyleGAN is able to understand that both sides of the images are very different and respects that when generating content.
it works! The normal maps look nice and correspond to the diffuse features.
What if?…
The diffuse images generated with the image-pairs are a bit weak and uninteresting, because the source dataset isn’t as bright and variate as the DTD. But unfortunately the DTD doesn’t have normal maps associated. :(
I want a rich diffuse texture and a corresponding normal map… 🤔
Normal map generation from source diffuse texture
I had to find a wat to turn any given image into a somehow working normal map. To do this, I tested Pix2Pix (Image-to-Image Translation with Conditional Adversarial Nets) with the image pairs composed for the previous experiment.
I trained it locally with 790 images, and after 32h of training I had some nice results:
Pix2Pix generates consistent normal maps of any given texture.
Chaining models
Workflow idea:
Diffuse from StyleGAN -> input to Pix2Pix -> generate the corresponding normal map
First I had to brig the Pix2Pix trained model to Runway. [following these steps].
Then I used StyleGAN’s output as Pix2Pix Input. 👍
With the help of Brannon Dorsey and following this fantastic tutorial from Daniel Shiffman, we were able to run a P5js sketch to generate randomGaussian vectors for StyleGAN, and use that output to feed Pix2Pix and generate the normal-maps.
Final tests / model selection
From all the tests, the learnings are the following:
Stylegan for textures works good - [SELECTED] - dataset = 5400 images
StyleGAN for normal maps works good, but there’s no way to link the generated maps to diffuse textures - [DISCARDED]
StyleGAN trained with a 2-in-one dataset produces nice and consistent results - but the datasets used did not produce varied and rich textures. [DISCARDED]
Pix2Pix to generate normal maps from a given diffuse texture works good. [SELECTED] - dataset = 790 image pairs
Pix2Pix trained with 10000 images produced weak results. [DISCARDED]
The final approach is: StyleGan trained with textures from the DTD dataset (5400 images) -> Pix2Pix trained with a small (790) dataset of Diffuse-Normal pairs from cc0Textures
Visualization & Interaction
I really like how runway visualises the latent space using a 2D grid to explore a multi-dimensional space of possibilities. (in resonance with some of the ideas in this article: Rethinking Design Tools in the Age of Machine Learning ).
It would be great to build a similar approach to explore the generated textures within a 3D environment.
To visualise textured 3D models on the web, I explored Three.js and other tools.
babylonjs seems the best suited for this project.:
A quick test with the textures generated from StyleGAN looks nice enough.
Trying to add the grid effect found in runway makes the thing a bit slower but interesting. The challenge is how to change the textures on-the´fly with the ones generated by StyleGAN/Pix2Pix…
After some tests and experimentations I managed to load video textures, and display them within a realtime web interface. 👍
mouse wheel/pinch= zoom
click/tap + drag / arrow keys = rotate
*doesn't seem to work with Safari desktop
Learnings & Release
The StyleGAN behaved as expeced: producing more interesting results when given a more rich and varied dataset.
Pix2Pix model has proven strong enough, but results could be better. For next iterations of this idea I should explore other models. like MUNIT or even some StyleTransfer approach.
Both checkpoints have been released and are free to use at Runway. StyleGAN-Textures + Diffuse-2-Normal
You can also download the model files here for your local use.
Thanks & next
This project has been done in about two weeks, within the Something-in-Residence program at RunwayML. Thanks.
I’ve learned a lot during this project and I’m ready to build more tools and experiments blending Design/AI/3D/Crafts and help others play with ML.
For questions, comments and collaborations, please let me know -> contact here or via twitter.
Background & Glossary
Some impressive work has been previously done in that area:
Some prints:
confusing coleopterists / 🤔🐞
breeding bugs in the latent space
breeding bugs in the latent space
A beetle generator made by machine-learning thousands of #PublicDomain illustrations.
Inspired by the stream of new Machine Learning tools being developed and made accessible and how they can be used by the creative industries, I was curious to run some visual experiments with a nice source material: zoological illustrations.
Previously I ran some test with DeepDream and StyleTransfer, but after discovering the material published at Machine Learning for Artists / @ml4a_ , I decided to experiment with the Generative Adversarial Network (GAN) approach.
Creating a Dataset
Through the Biodiversity Heritage Library, I discovered the book: Biologia Centrali-Americana :zoology, botany and archaeology, hosted at archive.org, containing fantastic #PublicDomain illustrations of beetles.
Through a combination of OpenCV and ImageMagick, I managed to extract each individual illustration and generate nicely centered square images.
Training a GAN
Following the Lecture 6: Generative models [10/23/2018] The Neural Aesthetic @ ITP-NYU, Fall 2018 - [ Lecture 6: Generative models [10/23/2018] -> Training DCGAN-tensorflow (2:27:07) ] I managed to run DCGAN with my dataset and paying with different epochs and settings I got this sets of quasi-beetles.
Nice, but ugly as cockroaches
it was time to abandone DCGAN and try StyleGAN
Expected training times for the default configuration using Tesla V100 GPUs
Training StyleGAN
I set up a machine at PaperSpace with 1 GPU (According to NVIDIA’s repository, running StyleGan on 256px images takes over 14 days with 1 Tesla GPU) 😅
I trained it with 128px images and ran it for > 3 days, costing > €125.
Results were nice! but tiny.
Generating outputs
Since PaperSpace is expensive (useful but expensive), I moved to Google Colab [which has 12 hours of K80 GPU per run for free] to generate the outputs using this StyleGAN notebook.
Results were interesting and mesmerising, but 128px beetles are too small, so the project rested inside the fat IdeasForLater folder in my laptop for some months.
In parallel, I've been playing with Runway, a fantastic tool for creative experimentation with machine learning. And in late November 2019 the training feature was ready for beta-testing.
Training in HD
I loaded the beetle dataset and trained it at full 1024px, [on top of the FlickrHD model] and after 3000 steps the results were very nice.
From Runway, I saved the 1024px model and moved it to Google Colab to generated some HD outputs.
In December 2019 StyleGAN 2 was released, and I was able to load the StyleGAN (1) model into this StyleGAN2 notebook and run some experiments like "Projecting images onto the generatable manifold", which finds the closest generatable image based on any input image, and explored the Beetles vs Beatles:
model released
make your own fake beetles with RunwayML -> start
1/1 edition postcards
AI Bugs a crypto-collectibles
Since there is an infinite number of potential beetles that the GAN can generate, I tested adding a coat of artificial scarcity and post some outputs at Makersplace as digital collectibles on the blockchain... 🙄🤦♂️
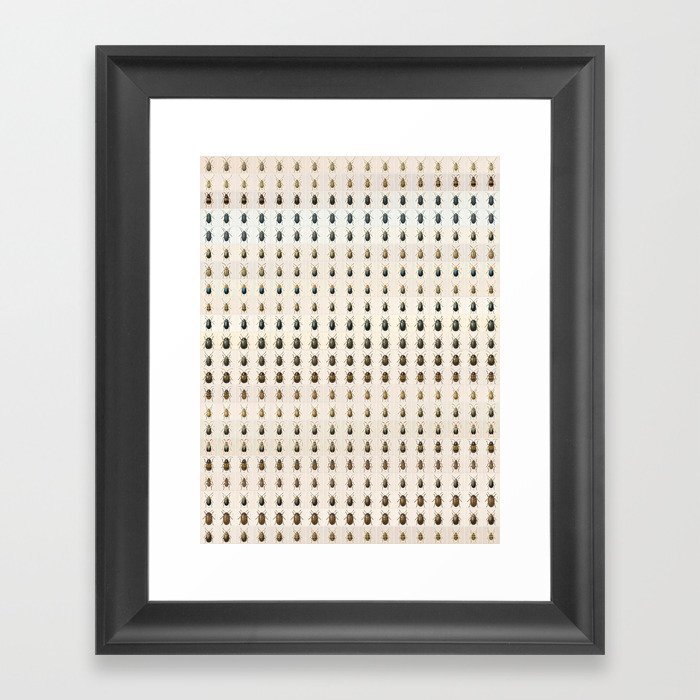
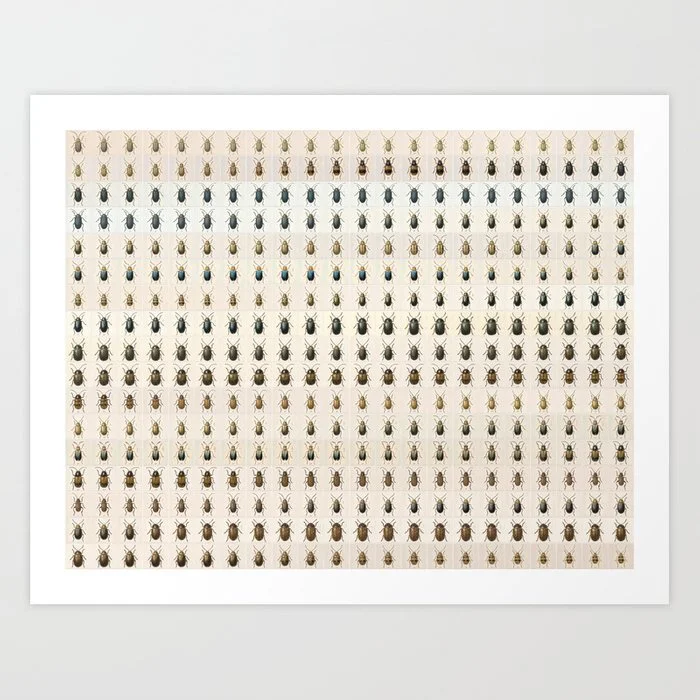
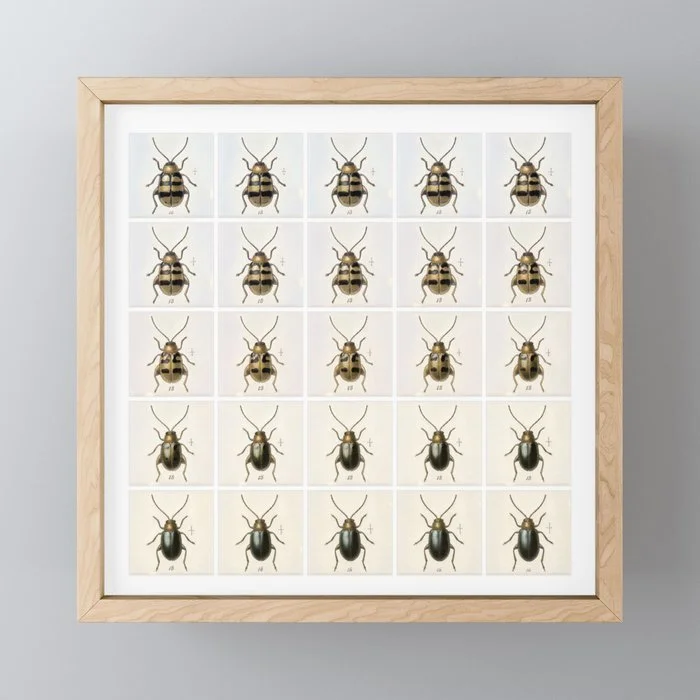
AI bugs as a prints
I then extracted the frames from the interpolation videos and created nicely organised grids that look fantastic as framed prints and posters, and made them available via Society6.
Framed prints available in six sizes, in a white or black frame color.
Every product is made just for you
Natural white, matte, 100% cotton rag, acid and lignin-free archival paper
Gesso coating for rich color and smooth finish
Premium shatterproof acrylic cover
Frame dimensions: 1.06" (W) x 0.625" (D)
Wire or sawtooth hanger included depending on size (does not include hanging hardware)
random
mini print
each print is unique [framed]
As custom prints (via etsy)
glossary & links
HEADFOAM
Design and test of shock-absorbing structures produced by AM to be applied to helmets used as PPE (Personal Protective Equipment) in sports as climbing, biking, skating, etc.
Shock Absorbing Structures For Sports Helmet
Head Protective Equipment Fabricated With Additive Manufacturing
Project: Design and test of shock-absorbing structures produced by AM to be applied to helmets used as PPE (Personal Protective Equipment) in sports as climbing, biking, skating, etc.
Innovation: Use of an impact-absorbing structure designed specifically for AM production.
Challenge: We believe that an inner structure of a safety helmet designed with a shock-absorbing lattice structure and produced by AM would make a better helmet.
Application: The solution PPE helmet can be used in outdoors sports as climbing or biking or skating..
Activities:
Design study on several techniques to generate lattice structures.
Dynamic Impact Tests
Test consisted in dropping 5 kg round metal object onto the test subject from a height of 1m. Test was run at the User Partner lab, equipped with testing machinery, using the 1011e 1012_MAU 1002_2W ALU_SF equipment by CKL engineering (figure 3 left). Machine measures the total impact force which is absorbed by the sample, measured in Newtons.
Test results evaluation
Helmet inner part designed with shock absorbing lattice
prototypes & tests
LaaS / Lattice as a Service
This project has received funding from the European Union’s H2020 Framework Programme for research, technological development and demonstration under grant agreement no 768775.
Project supported by AMable and I4MS, done In collaboration with: ProductosClimax S.A.
AMable [AdditiveManufacturABLE ] provides support to SMEs and mid-caps for their individual uptake of additive manufacturing. Across all technologies from plastics through polymers to metals, AMable offers services that target challenges for newcomers, enthusiasts and experts alike.
I4MS -> The EU initiative to digitalise the manufacturing industry
SheDavid
3DScan Mashup / Venus de Milo + Michelangelo’s David
3DScan Mashup
Venus de Milo + Michelangelo’s David
Sculpture / 3D Printed in StainlessSteel
Source 3D models by @Scan_The_World initiative: Venus de Milo at The Louvre, Paris / Michelangelo’s David, florence
mootioon.com
Curated stream of inspiring 3D & MotionGraphics.
mootioon -> 3D / MotionGraphics / Render / Loop / C4D -> stream
An experiment to create a custom TV channel with an endless stream of inspiring motion graphics, 3D, vfx and CGI clips.
Inspired by the weird and mesmerising short-clips that inundated MTv during the 90’s, I always wanted a TV channel full of that, so I built one.
A script continuously finds clips from several sources
OBS to streams a folder containing the clips, randomly
The site links to most of the identified artists.
The service built for fun, and it doesn’t make money.
Agencies
Use 3D Printing in your next project: designing and preparing 3D models for 3D printing.
3D Print for Creative Agencies & Studios
Use 3D Printing in your next project, we help you by designing and preparing 3D models ready for 3D printing.
🛠 Problem: You want to use 3D Printing but don’t know how to start.
⚡️ Solution: We will assess if the project is doable or no and help you to make it real.
✏️ About us: We have extensive experience with 3D Printing in multiple projects.
🤔 You are an agency and got a fantastic project, and are thinking to use 3D printing: either to make a trophy for an event, create props for a photoshoot, or produce a short batch of items for a campaign.
Great idea! Probably everyone in the team has heard about 3D Printing, but most likely no one knows how to execute it :(
so, you might have some of the following questions:
- which materials shall we use?
- can we make something in colour?
- can we make something with metal?
- can we make something with ceramics?
- can we make something with flexible material?
- where to 3D Print?
- how expensive can it be?
You might have some talented designers on your team, and hopefully some master 3D modelling applications.
Sounds perfect, you should be ready to roll, but turns out that none of them have extensive experience with 3D Printing :( thus, there's a high probability that what they can design can't be 3D Printed due to constrains on the 3D Printing process.
So, your designers might have some of the following questions:
- how to create something to be 3D Printed?
- can I print multiple parts in one go?
- can my design have interlocking parts?
- what is the appropriate wall thickness?
- can the model be solid?
- shall the model be hollow?
- what is a "watertight" 3D model?
- how to remove manifold edges?
👍 We have extensive experience with 3D Printing in multiple materials, we have produced unique parts for clients, master pieces for molds, small batch series for product launches, personalised trophies, jewerly and ceramic products.
We will help you to assess if the project is doable or not. - for free -
Choose the appropriate material.
Design and 3D model for an optimal 3D printing.
Find a 3D Printing provider near you.
And help you produce the parts.
How it works:
You will work with a skilled product designer to create a design for your project.
We can start from scratch or evolve a product and re-design it for 3D Printing.
1 / Consultation / free
Assess if 3D printing is the best tech for your project.
-> Chat with us, or schedule a quick meeting to discuss your thoughts.
2 / Project Brief
Understand project goals.
Design inspiration.
Define materials.
Estimate production costs.
3 / Design phase
An expert 3D modeller will envision and prepare your design.
Iterative sessions where you will provide feedback in order to match expectations before production.
We will deliver a 3D file along with a final quotation of the production costs.
4 / Production & Shipping
3D Printed parts are made and delivered.
We will help you order the 3D Printed products with the 3D Printing bureau that best fits your needs.
Our production partners are in USA and EU, and serve globally.
What you get:
Up to 3 design iterations
Ownership of the digital 3D file.
Printability guarantee
*cost of 3D printing is not included
Approximate costs:
As a solution-based studio, we understand that price greatly depends on complexity of the problem to be solved, nevertheless, our experience creating designs for 3D Printing, allows us to set a tiered-price, that works in most cases:
3D Doctor:
> 1000 €
Projects where a 3D file is provided and needs minor tweaks to make it 3D printable (i.e Generate a valid geometry, thickness, hollow a model...)
Sketch to 3D:
> 5000 €
Projects where a clear idea, style and dimensions of the final object is drafted and needs to be modelled specifically for 3D Printing.
Concept to 3D:
> 9000 €
Projects where no prior design work has been done and ideation + design + modelling is needed.
Artificial Scarcity Store
A daily creation, temporarily available for purchase.
A random creation,
temporarily available for purchase.
Launched Today:
- available for few days, until the next update.
Too Late:
- not available anymore
What is this?
This is a design.store, you can buy what has been launched today, and it will be shipped to your door, like any other online store. *here the details
But beware, today’s item will be replaced by a new one tomorrow; and it won’t be available anymore, ever.
All items are unique to this site, and exclusively deigned by Bernat Cuni.
Mainly it is a store of things that can’t be bought, except for one.
It is an ongoing experiment on design, digital fabrication, consumerism, globalisation, social networks & niche markets.
The Artificial Scarcity Store is supposed to trigger different reactions:
Some, moved by a FOMO, might feel unconfortable knowing that today’s piece will become unavailable tomorrow.
Others, facing the paradox of choice, might feel uneasy with the thought that tomorrow’s piece could be better than today’s one, and are unable to make a decision.
I’m thankful to you, the ones who get it.
For me, the main driver is crativity.
The Artificial Scarcity Store is a daily challenge where to place my whatevers and sidekick ideas turned into buyable items. The ultimate reason to make them temporarily available is to not clutter my headspace, and have less things to manage.
Technically, I use a network of providers to fabricate and ship the goods.
A design can be turned into multiple items:
if it is a graphic that gets printed on a product, I tend to use Society6
if it is a 3D model, I usually use Shapeways as a production partner, or I might post it on Sketchfab if it is an asset that could be used by other 3D developers.
If it is digital content, like a DesktopWallpaper or a 3D model, I host it locally at this site, or I might use pay-links to make a file downloadable.
Nice?
I hope you enjoy the Artificial Scarcity Store, contact for any question/comment.
Store Details
Payments are securely processed and encrypted by Squarespace.
Delivery times is about 2 weeks (for US orders), add another week if ordering from the rest of the world.
Orders can be cancelled within 24h.
Customer service is a priority, if something goes wrong with the product we’ll try to solve it, and issue a partial or full refund accordingly.
All items are fabricated by 3rd parties.
XR Design
AR/VR experiences for brands and custom projects
Design for Mixed Reality
Exploring AR/VR tools for creativity and augmented experiences.
AR/VR projects brands and custom projects
Tools & Platforms
Augment your projects and make them available in multiple mediums.
iOS Ready - natively load 3D models and animations in AR
Unity - merge your content with a game engine
Dedicated Apps - enrich your project with a AR/VR app
Cross-platform - Oculus / Vibe / PlayStation VR
Creation in VR.
Experiment with the newest tools and creative software to create unique content.
Shareable
Publish 3D content on webBased galleries
Gallery
Explore some of our models
3D Scan
Creating a high detail digital archive of our material environment.
Using multiple 3D scanning technologies we can create high detail digital copies of our material environment.
HighRes scans for the creative industries, CGI, Gaming, AR/VR productions, educational content and cultural preservation.
High resolution texture maps,
HDLP - HighDefinition/LowPoly models: Manually retopologized from millions of polygons to an average of 5k-20k with HD NormalMaps.
Computer Graphics Imagery (CGI) reigns most of the contemporary media. (Did you know that H&M uses digital female 3D models on their website? and that what you see on the IKEA catalog is digitally crafted? and that the food that appears on commercials is also 3D?).
The omnipresence of CGI, combined with the rise of AR/VR platforms and the accessibility of 3D Gaming engines, creates the need for content.
We (humanity) made lots of stuff to ta date, and now, that stuff can be digitally captured and archived for digital re-use.
Some samples of models captured with photogrammetry:
TreeBark scanned to design the TreeRing / here a making-of tutorial
Experiments
Design & Software Solutions for Additive Manufacturing
Design & Software Solutions for Additive Manufacturing
Additive Manufacturing allows for creative fabrication of unique parts and prototypes.
Designing specifically for this technology we can make impressive products suited for multiple applications.
Example:
meta.materials
Designing material behaviour through internal structures.
Lightweight: Reduce volume.
Custom Performance: Variable density lattice.
Optimised for AM materials and manufacturing methods.
Project Sample:
Shock absorbing insole.
Biometric data-driven variable density lattice structure.
Design of a shock absorbing lattice structure.
Populate the insole volume with the designed structure.
Align the insole with a 3D scan of the wearer’s foot.
Define the performance of the insole by selectively assigning different density to the lattice structure.
Permutation
Generative collection, designed with code, 3D printed in Stoneware.
Generative collection, designed with code, 3D printed in Stoneware.
Pieces composed by the random combination of nine basic units, placed around a cylinder.
Each piece is unique and exists within an immense landscape of millions of possible different combinations.
Material: Stoneware
Printed by: bcn3Dceramics
Software: Rhino3D / Grasshopper
Machine: PotterBot 3D printer
Design by: Bernat Cuni
Exhibited at: Argillà Argentona - International ceramics fair
P114.3
148.791.629.670.981.130.805.037.453.479.575.340 possible combinations.
One hundred and forty eight decillion, seven hundred and ninety one nonillion, six hundred and twenty nine octillion, six hundred and seventy septillion, nine hundred and eighty one sextillion, one hundred and thirty quintillion, eight hundred and five quadrillion, and thirty seven trillion, four hundred and fifty three billion, four hundred and seventy nine million, five hundred and seventy five thousand, three hundred and forty.
P16.4
66.305.137.490.523.096 possible combinations.
sixty-six quadrillion, three hundred and five trillion, one hundred and thirty-seven billion, four hundred and ninety million, five hundred and twenty-three thousand, one hundred and four
art.faces - AI sculpts 3D faces from famous paintings
Exploration on face detection and 3D reconstruction using AI
Exploration on face detection and 3D reconstruction using the "Large Pose 3D Face Reconstruction from a Single Image via Direct Volumetric CNN Regression" code by Aaron S. Jackson, Adrian Bulat, Vasileios Argyriou and Georgios Tzimiropoulos.
We selected 8 Famous Paintings and let the Convolutional Neural Network (CNN) perform a direct regression of a volumetric representation of the 3D facial geometry from a single 2D image.
Mona Lisa
Leonardo da Vinci
c. 1503–06, perhaps continuing until c. 1517
Oil on poplar panel
Subject: Lisa Gherardini
77 cm × 53 cm
Musée du Louvre, Paris
Girl with a Pearl Earring
Meisje met de parel
Johannes Vermeer
c. 1665
Oil on canvas
44.5 cm × 39 cm
Mauritshuis, The Hague, Netherlands
The Birth of Venus
Nascita di Venere
Sandro Botticelli,
The Birth of Venus (c. 1484-86).
Tempera on canvas.
172.5 cm × 278.9 cm.
Uffizi, Florence
Las Meninas
Diego Velázquez
1656
Oil on canvas
318 cm × 276 cm
Museo del Prado, Madrid
Self-Portrait
Vincent van Gogh
September 1889
Oil on canvas
65 × 54 cm
Musée d'Orsay, Paris.
This may have been Van Gogh's last self-portrait.
Laughing Cavalier
Frans Hals
1624
oil on canvas
83 cm × 67.3 cm
Wallace Collection, London
American Gothic
Grant Wood
1930
Oil on beaverboard
78 cm × 65.3 cm
Art Institute of Chicago
The Night Watch
Rembrandt van Rijn
1642
Oil on canvas
363 cm × 437 cm
Rijksmuseum, Amsterdam
The technology behind:
Code available on GitHub
Test your images with this demo,
This tech is fantastic and amazing, but same as with DeepFakes, it should be handled with care… Once we can generate accurate 3D representations of anyone with minimal input data, there’s the possibility to use that 3D output as digital identity theft.
As well questioned in this VentureBeat article :
So what happens when the technology further improves (which it will) and becomes accessible to marketers and brands (which it always does)? Imagine a casting call where a dozen actors are digitally and convincingly superimposed on a stand-in model prior to engaging the actors in real life.
We can imagine a scenario where videos are created using someone’s face (and body?) to make them do whatever they want, without consent.
On the other hand, AI is getting so smart, that in some cases, it doesn’t need a real input to go wild and imagine possible realities.
In this paper by NVIDIA, a generative adversarial network is able to picture imaginary celebrities.
Particularly in the 3D space, we see a huge potential for this kind of technology.
Digital Ceramics
Ceramic 3D Printing products and services for the industry & the arts
Ceramic Additive Manufacturing products and services for the Industry & the arts
Powder Binding / SLA / Paste Extrusion
3D Scan / cultural heritage
12th Century Capitals 3D scanned & released.
Capturing architectural details with photogrammetry.
12th Century Capitals 3D scanned & released.
Capitell Arnau Cadell @ Monestir de Sant Cugat
Flint, Hive & Bubbles
A generative 3D Printed ceramic tableware.
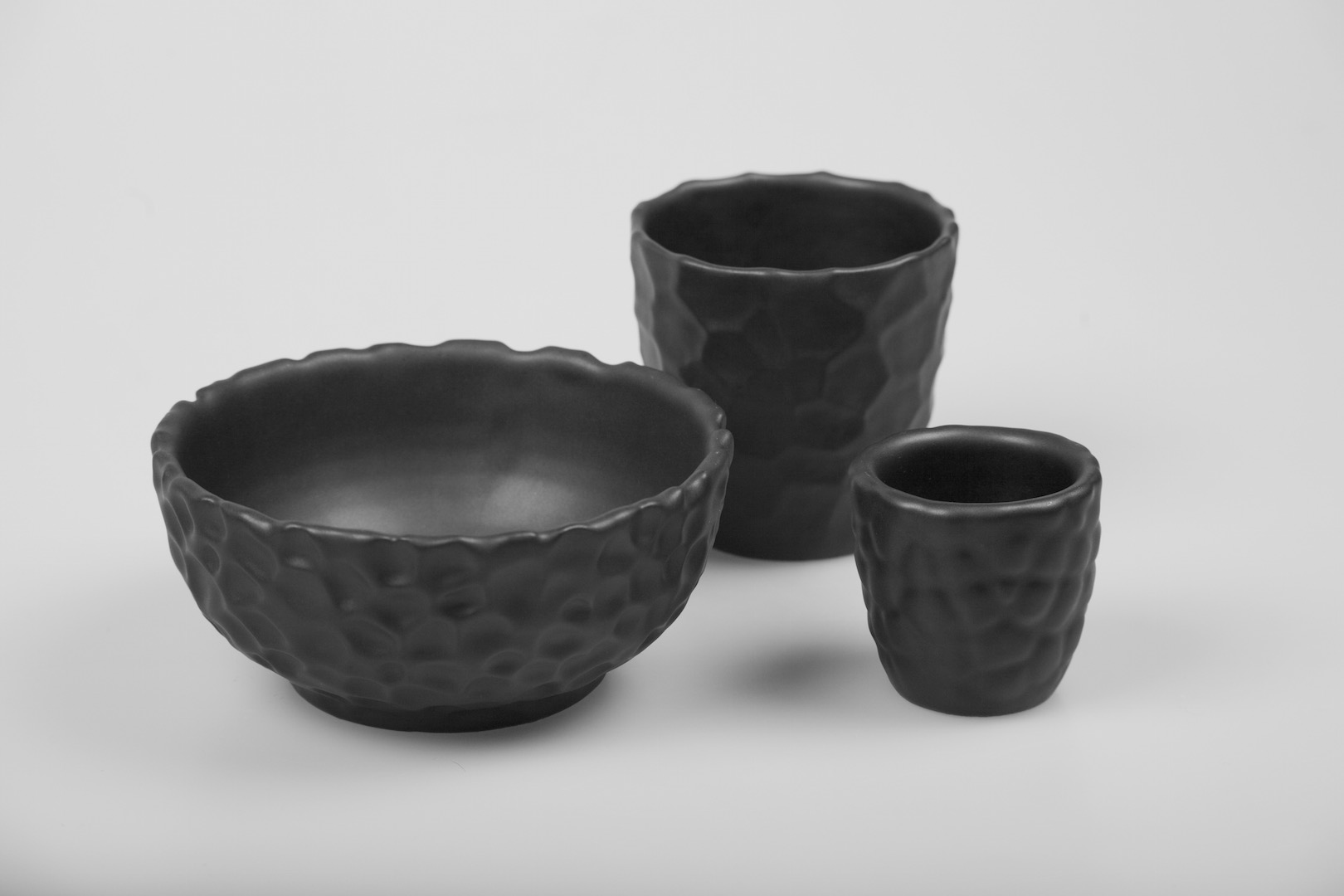
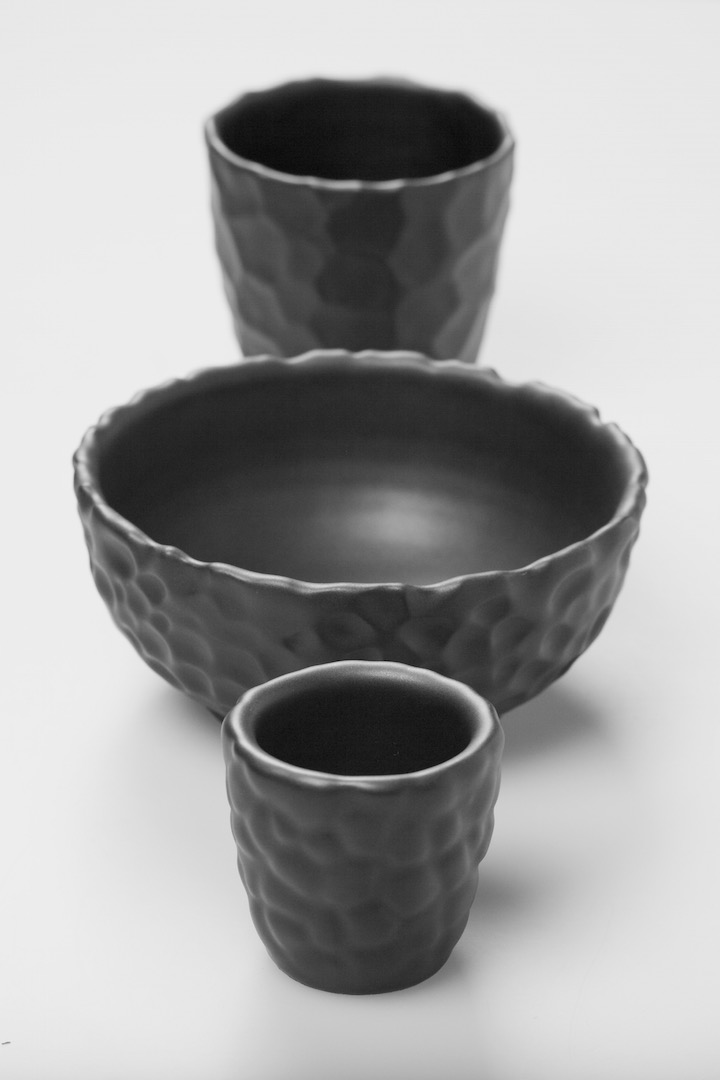
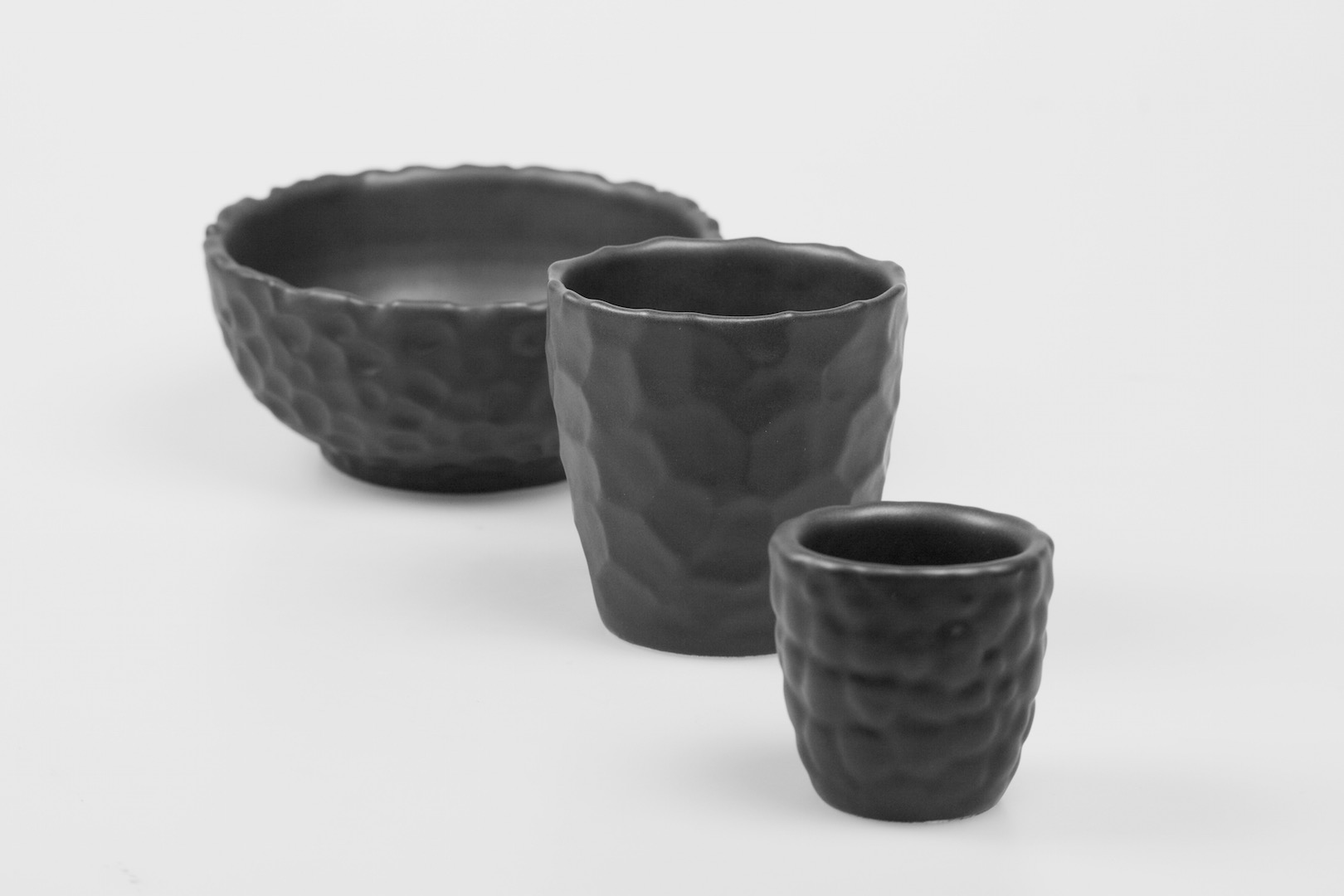
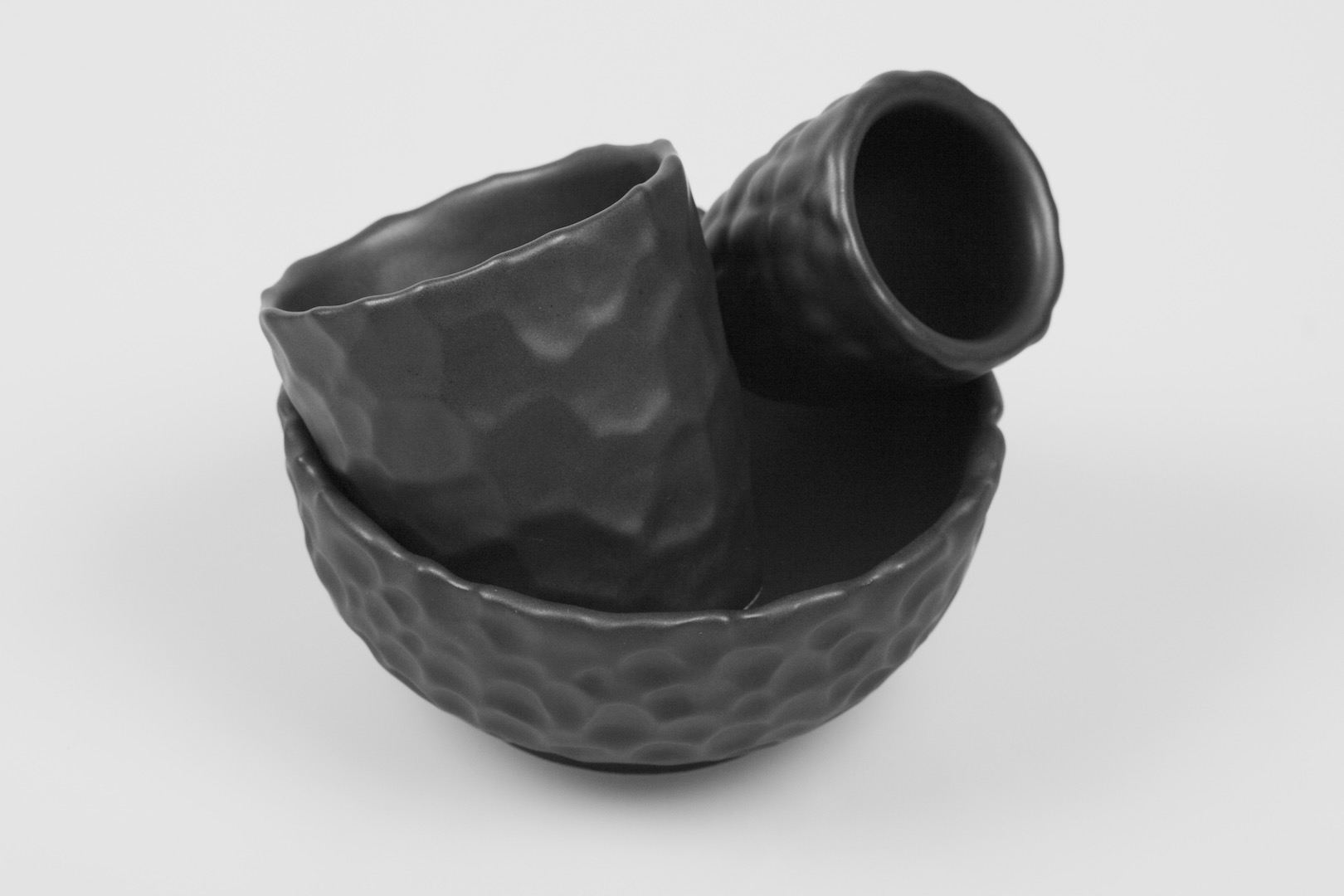
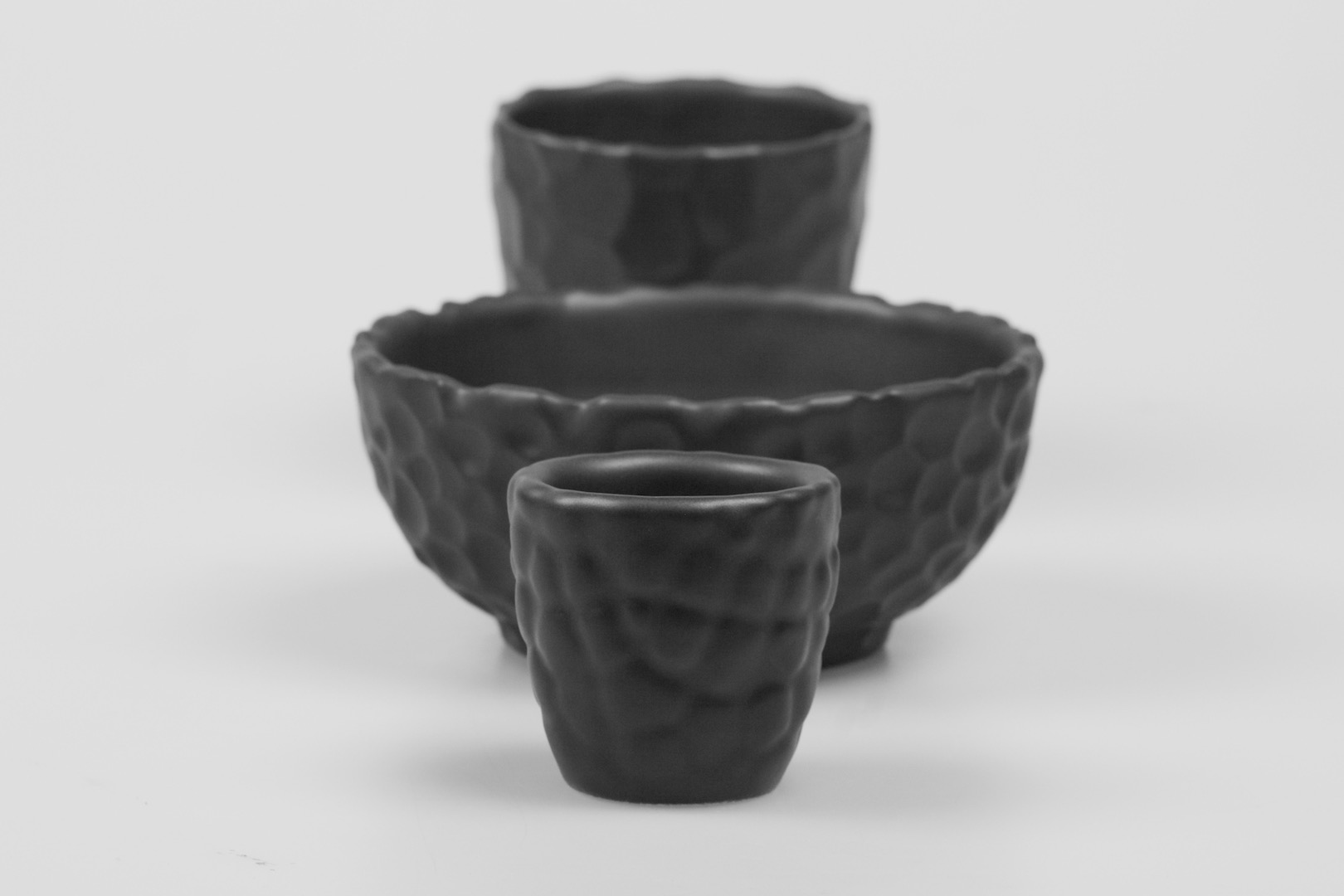
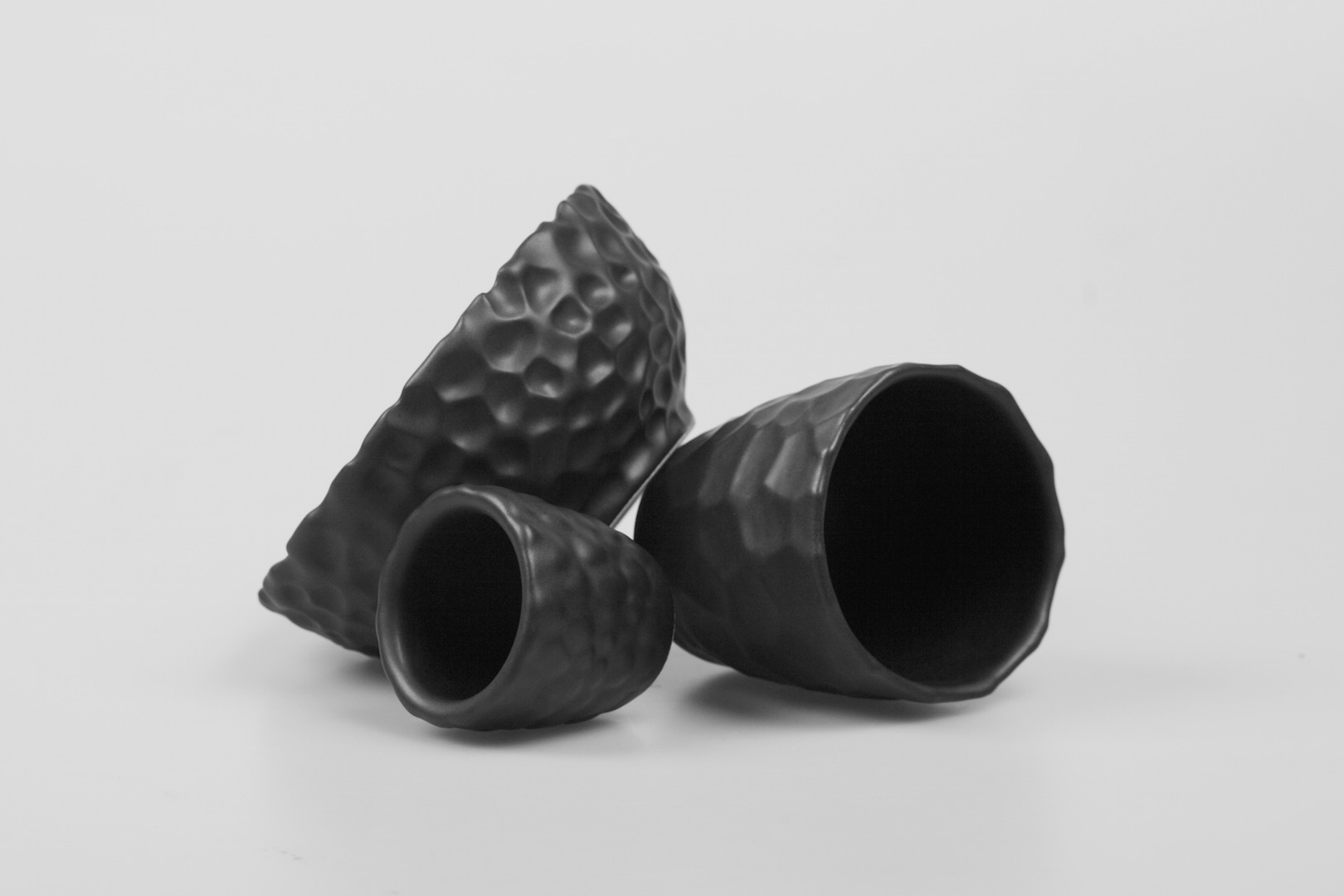
One design, unlimited variations.
Designed with Symvol for Rhino for Uformit.
DESIGN YOURS:
nicetrails
Nicetrails was a service to turn GPS tracks into tiny mountains, 3D Printed - discontinued
Turn GPS trails into tiny mountains, 3D Printed
nicetrails.com is a tool for anyone to visualize and share gps-geolocalized data in 3D.
EOL / 2016-2022
nicetrails service is discontinued.
We started this project in 2016 building a custom software to turn GPX tracks into 3D-printable files; now, in 2022 the internet has changed, and nicetrails should update its web usability and engine speed… but our focus is currently in another directions… so, discontinuing seems like a good choice now.
Shutting down is a bit sad, but we enjoyed the journey.
Thanks for your support during all this time.
Bernat / designer
Oscar / developer
Website Archive
Backend Archive / 3D engine / interface
Some models created
Alternatives
Here some alternatives to keep turning GPS to 3D:
For large scale 3D Prints: WhiteClouds-Terrain
For 3D Artists: BlenderGIS addon
For a web app: Maps3D
3D Printed Figurines from Children’s Drawings
CrayonCreatures
A service to turn wonderful drawings into awesome figurines; nice looking designer objects to decorate the home and office with a colorful touch of wild creativity.
CrayonCreatures.com / (discontinued)
Turning children’s drawings into 3DPrinted figurines.
Children drawings are weird and beautiful.Kids produce an immense amount of drawings that populate fridges, living rooms and workspaces of parents, family & friends.Those drawings are amazing.
Now you can turn those drawings into volumetric figurines; nice looking objects that will decorate your home and office with a colorful touch of wild creativity.
http://CrayonCreatures.com will bring to life the kid’s artwork by 3D modeling a digital sculpture and turn it into a real object using 3D Printing technology.
The figurines are custom designed and digitally crafted one by one.
Material: full color sandstone | Size: about 100mm tall.
End of Life / 2013-2023
One Coffee Cup a Day | 30 days, 30 cups
One Cup a Day project is an experiment on creativity and rapid manufacturing, by ideating, designing, modeling and making available for production and purchase a coffee cup within 24 hours, everyday during one month.
One Cup a Day project is an experiment on creativity and rapid manufacturing,
by ideating, designing, modeling and making available for production and purchase a coffee cup within 24 hours, everyday during one month.
The project was exhibited at the Ceramic Event VII in Brussels.
By the end of each day, a new espresso coffee cup was made be available for sale here.
The cups are printed in Glazed Ceramics by Shapeways & i.Materialsie.
*the following images are computer generated visualizations of the cups, not the actual objects.
As 3D-printing in ceramics is a novel and experimental process, the 3D-printed cups might look different than the images below.
Design one yourself
This project is open for collaboration,
Here I make available the source files (as .STL & .IGS files of a template basic cup,
please, feel free to play around and modify it.
3D Print it yourself
The OctoCup is shared at Thingiverse, feel free to grab the files, experiment with it and share the results.
I printed one with a RepRap and it looks nice, (useless, but nice).
Learnings
done! I made it; Design 30 cups in 30 days,
They are all at the store, ready to be 3DPrinted on demand.
Surprisingly, the idea generation has been the easiest part of the project,
I still have tons of cup ideas on my head that were not designed: (Banana Handle cup / Toilet cup / Matrioska cup / Melted cup / Mustache cup…)
The hardest part of the project has been preparing the 3D geometries and files for 3DPrinting.
The 30 designs can be grouped into 3 categories
Morpho-jokes = cups adopting shapes from other contexts with a funny result.
Uselessly nice = cups whose striking uselessness makes them interesting.
Texture Analogies = cups wrapped by textures.
I really enjoyed the project format, the challenge and the results.
3D Model vs. 3D Print:
The first 3DPrinted Cup in Ceramics just arrived,
and it looks fantastic!
The glazed layer is quite thick and it hides a lot of the original detail, which is OK for the OctoCup, but it might be a bit too soft for other designs, like the Knitted Cup or the LowResolution Cup.
It has an overall look and feel of a hand-made product, which is very interesting, because this object was “untouched by humans” (except for finishing and shipping), meaning that it was designed in computer, with perfect radiusess, and perfect proportions, Then, it was 3DPrinted with a computer controlled machine accuracy, but the result is bumpy and imperfect, and it is nice.
It almost feels like an craft object, but this cup is entirely a computer-born and grown object.
Update: Shapeways improved their 3DPrinting process and the results are much better now.
Notes
About the design:
The cups are designed as a creative exercise and as a proof of concept for digital fabrication, in order to achieve something unthinkable some time ago: Create a product from the idea to the consumer in less than 24 hours.
Some designs are compromised due to time constrains, but that’s OK.
Not all the cups are ment to be the best and most functional of all coffee cups ever, they are designed with style, fun and diversity on mind.
About the material:
The coffee cups are 3D Printed in Glazed Ceramics.
Glazed Ceramics are food safe, recyclable and heat resistant. The glaze is heatable up to 1000 degrees celcius, at which point the glaze will start to damage.
3D Printed Glazed Ceramics material properties are exactly the same as standard ceramics as it is produced with fine ceramic powder which is bound together with binder, fired, glazed with lead-free, non-toxic gloss finish. For some designs with clear bottoms, the bottom side may remain unglazed.
Glazing reduces definition of design details, for example grooves will fill with glaze. up to 1 mm of glaze can be added in certain areas.This means that some cups might look much smoother once printed than how they look on the drawings, keep that in mind if you purchase any of them.
Step 1. 3D Printing, it takes about 4 hrs to print a 4 inch piece. During this step, binder is deposited on a bed of ceramic powder. After one layer is done, a new layer of ceramic powder is spread on top and binder is again deposited on that layer.
Step 2. After all the layers are printed, the box of ceramic powder is then put in an oven to dry, the models in the box solidify during this process.
Step 3. After the models solidify, the models are taken out out of the box and depowdered. During this stage, the models are solid but in a brittle, fragile state.
Step 4. Afterwards, the models are fired in a kilm with a “high fire.” This firing will lock in the structure of the model. After this step, a base model is created. The surface finish is very rough.
Step 5. Pre-glaze coating spray, a water based spray, is then applied. Then the models are fired away in a kiln at a lower temperature. Afterwards, the model is a bit more smooth and ready for glazing.
Step 6. The glaze spray is applied, and the models are again fired in the kiln. After this process, the model becomes shiny and smooth.
Note to buyers:
All cups are available for purchase here:
The cups are espresso size; about 65mm tall.
They might look quite small.
The images in this page are computer generated, not photos from the real cups.
3DPrinting in Ceramics is a novel and experimental production process, the resulting objects might loose detail and look different than the above images.
Production: Orders, payment, producing-printing, quality control, delivery & customer service processes is handled by Shapeways.com, a 3rd Party Company not related to Cunicode.
Pricing: 3DPrinting in Ceramics is quite expensive, that’s why the cups are very small, to keep the price down. Cunicode gets $5 per cup sold only, the rest goes to the producing company,
Further development
Thanks for the interest and support,
special thanks to ignant.de / Swiss-Miss / Neatorama / BoingBoing & others fantastic sites for writing about the project.
I'd like to 3DPrint all the cups and prepare an exhibition.
Please contribute to make it happen.
Thanks.